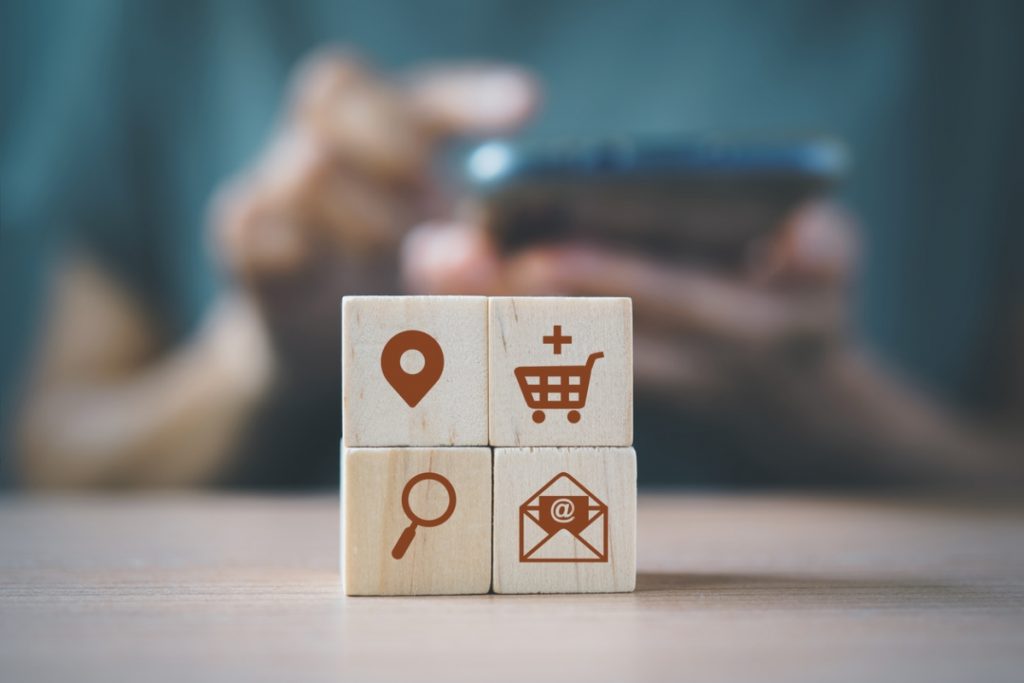
While almost everyone in eCommerce seems to agree that personalization is the future of digital commerce, actually doing it is another matter. There are lots of reasons for this, most of which revolve around time, resources and a knowledge gap.
But personalization is not only the preserve of the big eCommerce players. You can get started with personalized product recommendations using data you already have. Plus a small budget. You could also work with a trusted partner like MAQE…but we will talk about that later.
Personalized product recommendations are an overlooked CRO/personalization tactic. So we are going to tell you why you should use personalized product recommendations. What benefits they can bring to your business and how you can get started.
We hope this post will make personalized product recommendations a little less scary and a bit easier to understand.
Let’s get started.
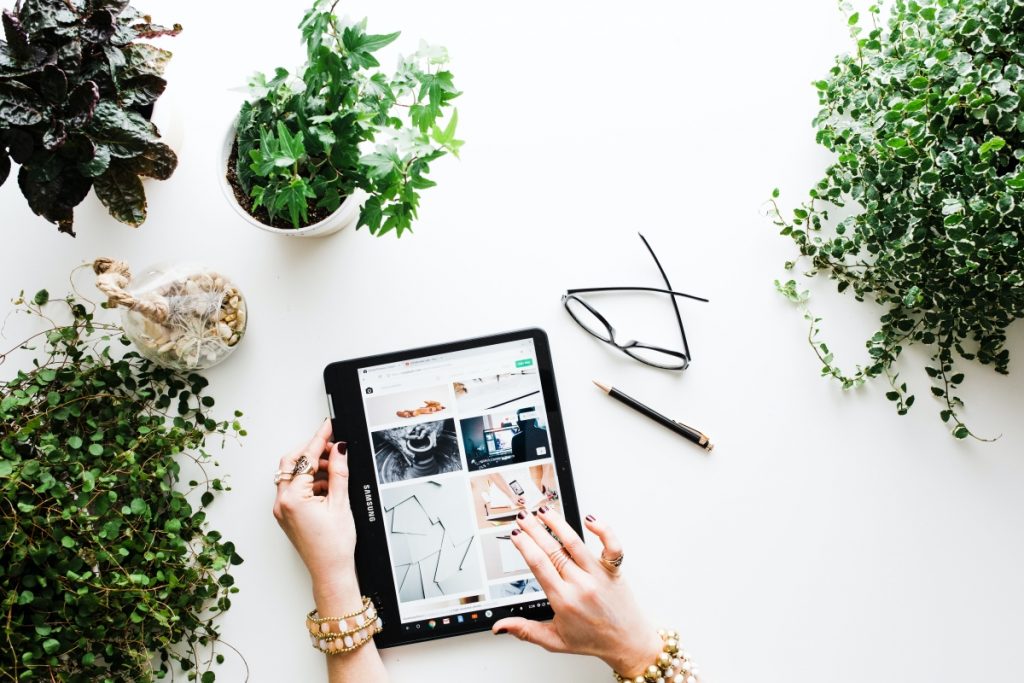
So…What Are Personalized Product Recommendations?
It is important to note that not all product recommendations count as personalized product recommendations.
Personalized product recommendations are when a site or app shows product recommendations that are unique to the individual user. This can be based on their behaviors, profile, location and purchase history. Machine learning algorithms power personalized recommendations. In fact, several algorithms working in tandem often produce the recommendations you see.
Some examples of personalized product recommendations include:
- Recommendation of products based on the individual users’ purchase history
- Recommendation of products based on the individual users’ location
- Recommendation of products based on the individual users’ browsing habits and history
- Recommendation of products based on topical, semantic and affinity relations between different products (eg. books by the same author or on the same subject, products that are often purchased together)
Product recommendations that are NOT personalized product recommendations are:
- Recommendation of products based on social proof (eg. popularity or strong reviews)
- Recommendation of products based on business goals or needs (eg. items on seasonal sale, low in stock, discounted products)
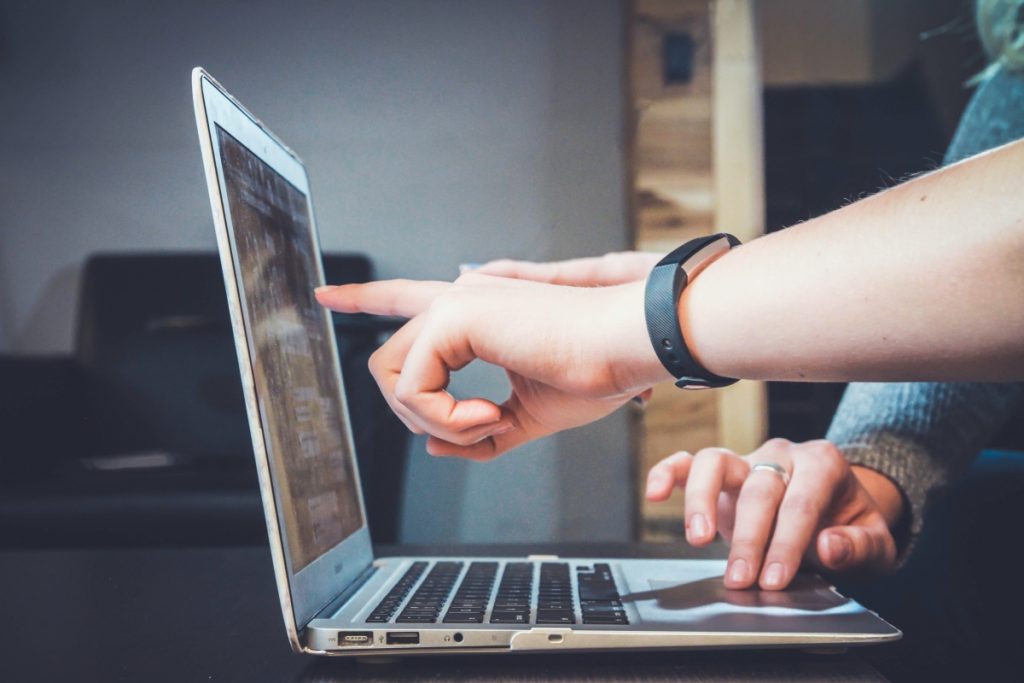
Why Should You Use Personalized Product Recommendations?
Any company that does business online should be looking at personalization strategies. Whether you are involved in B2C eCommerce or B2B service provision; personalization can improve your ROI and Average Order Value (AOV).
This is because customer needs and expectations have changed. According to Salesforce, 52% of customers expect personalized offers. This trends across all of internet commerce with 62% of customers say that their experience with one industry influences their expectations of others.
So even if you are a very niche B2B company, a lot of customers will expect you to be as capable as a brand like Amazon. Which is tough as most companies do not have the time or resources that Amazon has.
So where should you start? You have a few options available depending on your specific circumstances.
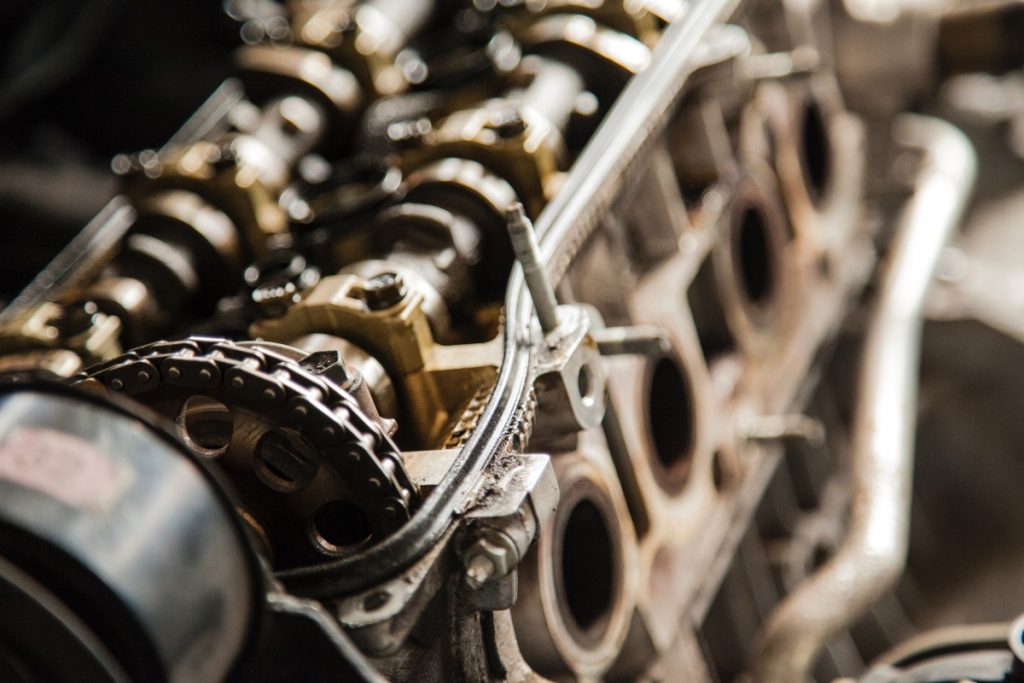
Personalized Product Recommendation Engines
The easiest way to implement personalized product recommendations is to use a recommendation engine or work with an external partner. But before getting down to the business of choosing your recommendation engine, you need to look at the data you already hold on your customers, if you even do hold any data.
You can start from scratch, that is always an option. So do not worry if you do not hold any first-party data. It will just take longer for your recommendation engine to fully optimize and deliver good results.
But for the best results long term you should implement a secure first-party data strategy. MAQE can help you with this. We can align your data and business needs so if you do implement a recommendation engine, you will have a solid data set to start with. Sorting data so it’s actionable and useful is a time-consuming process, so we would love to help you! Get in touch via [email protected] to discuss your needs.
There are lots of recommendation engines around, like Dynamic Yield, Recombee and Clerk.io. You could even A/B test them with an external partner (like MAQE hint hint) to see which one works best for your customers.
Can You Build Your Own Engine?
If you have the resources and the technical expertise to build your own product recommendation engine, then it is a great option.
By building your own solution you can create a recommendation engine that is tailored to your needs. And the needs of your customers.
However the process is very resource intensive, time consuming and messy. MAQE can help you to quickly build a custom recommendation engine solution that is unique to your business. Freeing up your development resource to work on more business critical projects.
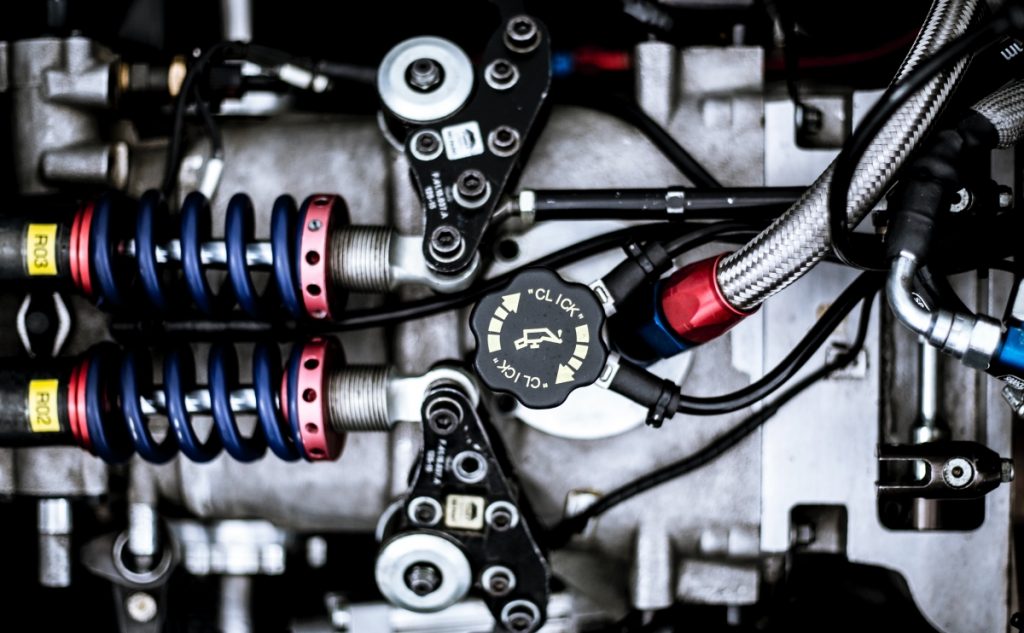
How Do Personalized Product Recommendation Engines Work?
This is a question that can lead to some very complicated, hardcore data science! But we will try to keep it as simple as possible here.
Most Recommendation Engines you have used, like Netflix, Spotify or Amazon work on the same principles. One aspect of these systems is recommender systems.
There are 3 basic types of recommender systems:
Content-based Filtering
A content-based filtering system uses user reactions to make recommendations to a user. The more a user reacts positively to certain products, the more the system can make guesses as to what products that user would like.
For example, say Netflix or YouTube were pure content-based filtering systems. When using those services, say you then clicked “thumbs up” on every comedy movie you see; In this instance the systems would then keep recommending comedy movies.*
Content-based filtering does not require other users’ data to make recommendations to one user.
*This is not how Netflix or YouTube actually work.
Collaborative Filtering
Collaborative Filtering is what you tend to see on eCommerce sites like Amazon, Shopee and Lazada.
Like content-based filtering, collaborative filtering systems use positive user signals to make recommendations. But collaborative filtering also uses data from other users to make recommendations.
For example you visit Lazada for the first time and the recommendations are very broad. But if you kept looking at Laptops for a long time it would then recommend more laptops. It would also recommend other items that users similar to you have reacted positively to. Using our laptop example, if you are buying an expensive laptop, you might want a case for it too. The system would handily show you lots of nice new laptop cases other users have bought.
This system can also use implicit feedback (clicks, search queries, purchases) and/or explicit feedback (review ratings, likes, dislikes) to make recommendations too.
Hybrid Filtering System
You may have made an educated guess that a Hybrid Filtering System is a mix of both of the above systems. And you would be right!
For sites and apps that hold a lot of user data, a hybrid system is usually the ideal filter system. It can gradually build profiles for new users and make solid recommendations for returning users.
There is a lot at play beyond these three types of recommender systems in a personalized product recommendation engine. For more technical information on this (and other data science topics) check out https://towardsdatascience.com/.
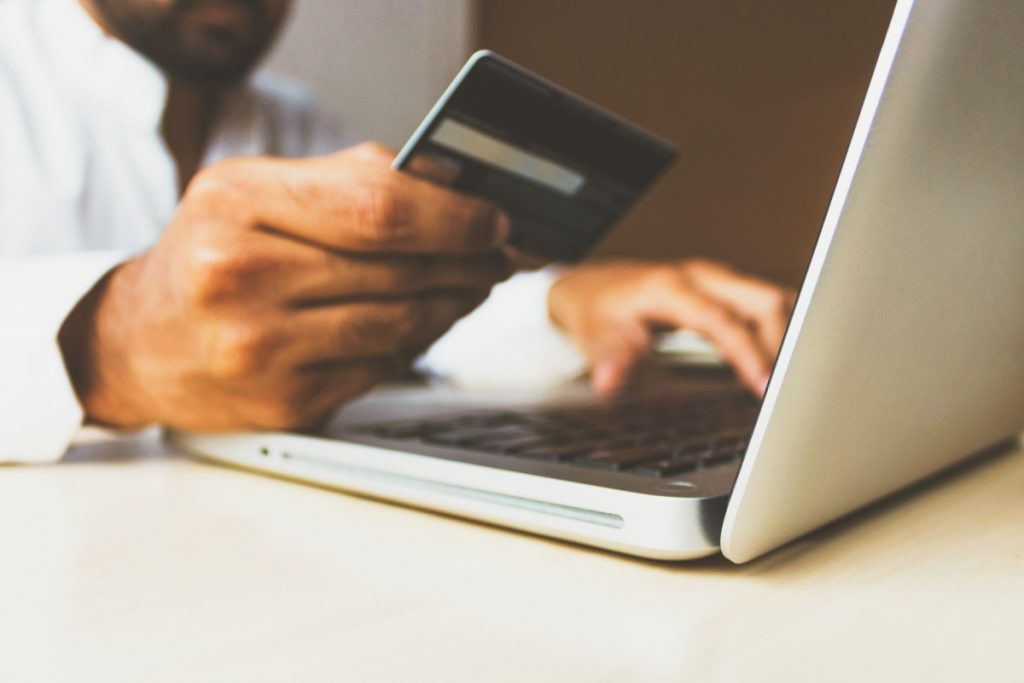
Where You Should Be Making Recommendations
Where should you be putting your amazing personalized recommendations?
Here are some key areas you focus on if you want to make personalized product recommendations on your commerce site.
Product page – This is THE best place for upselling and cross selling. Make sure you have recommendations on all your product pages
Home Page – Great recommendations on a home page can grab users’ attention. Which is vital if you want to convert first-time visitors into loyal customers. Get your best sellers and trending products into highly visible positions on your home page at the very least
Checkout/Cart pages – If you want to increase AOV then getting great recommendations onto your cart pages are a must. Next to product pages, cart pages are a perfect opportunity to upsell users by recommending something they would be interested in.
404 pages – The humble 404 page is an underutilized tool in your customer conversion toolkit. You do not want loads of 404 pages, but sometimes you cannot avoid them. So why not use this space to make recommendations based on your customer’s browsing history?
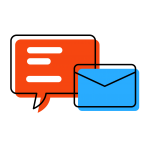
Talk to MAQE
Do you want to implement personalized product recommendations on your site but you are not sure where to start? Talk to MAQE. MAQE specializes in personalized commerce. So we will help you improve your AOV and conversion rates. Get in touch via [email protected].